🦋 Glasswing #6- Dangers of Open-Source LFMs; IP Paradox in AI
UK invests in BritGPT; Stanford replicates GPT like performance with Alpaca; OpenAI stays quiet about GPT-4 architecture
Welcome to 🦋 Glasswing! A weekly newsletter about AI research as it relates to its impact on human communication. Share with your friends and don’t that your secrets will be your Turing Tests. Thanks for reading!
1) Open-Source LFMs are inevitable
Open-Source Large Foundation Models (LFMs) are models that are developed, maintained, and distributed under open-source licenses, allowing free access, usage, and modification of the underlying code.
Most of the most well known LFMs today (e.g, OpenAI’s GPT-4, Google’s PaLM-E, Meta’s LLaMa, DeepMind’s Gopher) do not fall into the category of open-source LFMs. They are privately owned, capital-intensive, and have limited outside access or control of these models.
Previously, companies released open-source models with complete architectural and training details, such as Meta's OPT and Google's BERT. However, as models began exhibiting human-like communication abilities, the shift from "open" to "closed" AI arose, attributed by companies to safety concerns, but perceived by the public as a move to capitalize on their newfound potential.
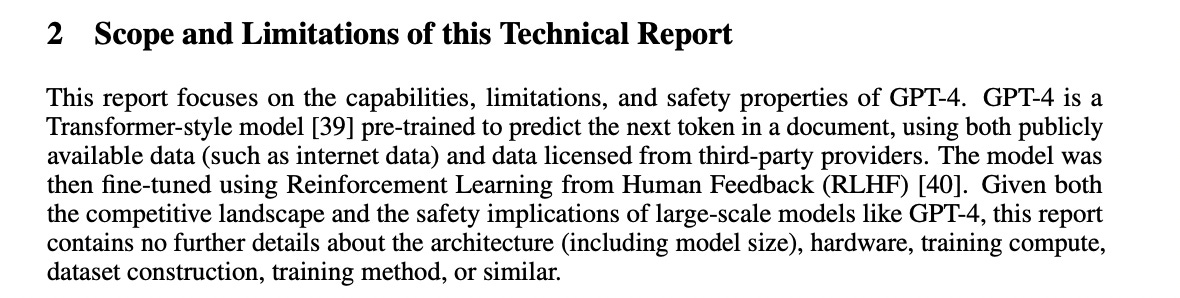
Industry labs like OpenAI have reshaped public perception of artificial intelligence, raising concerns about their creators' influence over the knowledge economy. The prevalent culture among these leaders, often rooted Effective Altruism, may not always align with the public's best interests when determining a model's merits or drawbacks.
Amidst such concerns, national impacts are becoming evident, as exemplified by the UK's £900 million investment in developing their own GPT model. This potentially reflects an “arms-race mentality” to reduce reliance on the US or China, indicating a shift towards federal labs investing in AI, rather than solely relying on corporate labs in the US.
1.1) Can’t Protect Your AI: Case Study with Meta LLaMA and Stanford Alpaca
Even if these corporate and government funded labs do manage create their own version of their LFMs, there is no guarantee that these models can’t be stolen, repurposed and used by bad-actors. I will use the case study with Meta and Stanford to demonstrate this.
Stanford researchers combined LLaMA (Meta’s LFM) neural network weights with input/output (I/O) data from a OpenAI’s commercial GPT-3 model to create a cost effective model called Alpaca with performance comparable to GPT-3 for a cost of $600. The neural net weights to LLaMA were leaked on 4chan.
What does this mean for open-source LFMs?
LLaMA has now become the benchmark for online capabilities, which is pretty scary. As a substantial advancement over previous open-source pre-trained models (such as OPT, BERT, and RoBERTA), LLaMA sets a new standard.
Combining LLaMA with I/O data from commercial models, such as GPT-4, implies that within its boundaries, it is theoretically possible for anyone to replicate a model akin to GPT-4. This case study highlights concerns regarding the dissemination and duplication of such models. Read more on the thread by Eliezer Yudkowsky.

Why would OpenAI be incentivized to release their model if any given customer could in theory replicate “near GPT-4 performance”? What power does this give to non-state actors or criminal organizations who may not respect legal restrictions? What are the legal restrictions?
2) Outcomes from Open-Source LFMs
What are the rules on using I/O data from OpenAI GPT-4 to improve a base-line trained model?
Their Terms of Use says that “You may not use output from the Services to develop models that compete with OpenAI”. This is why Alpaca from Stanford can’t be used for commercial purposes.
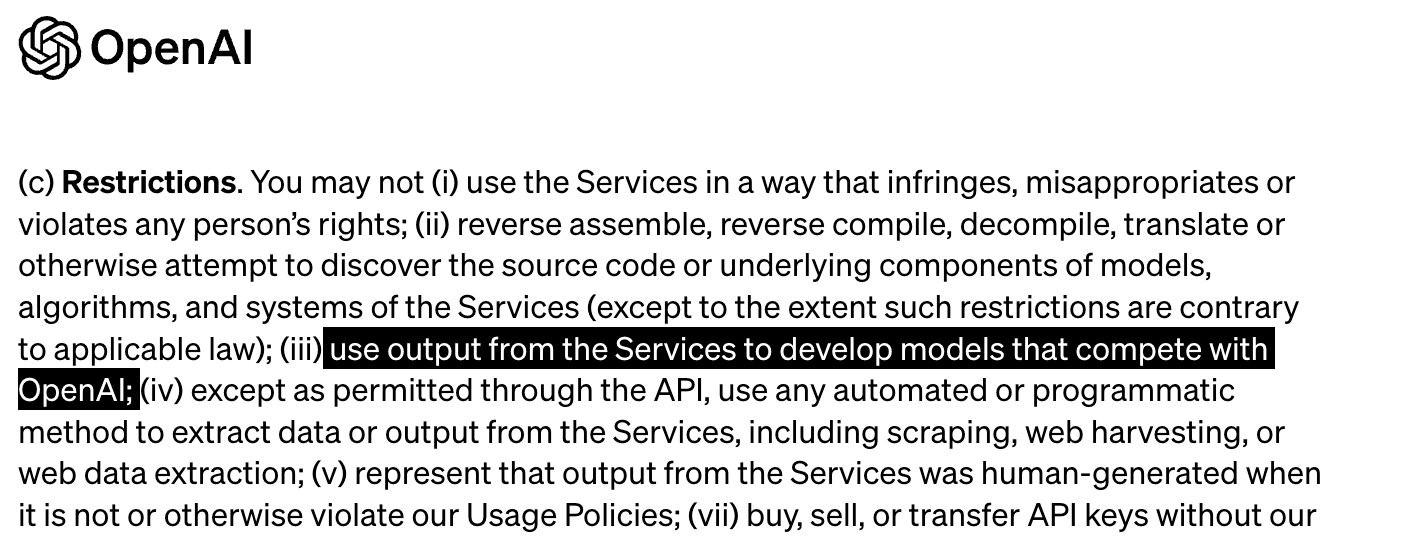
2.1) What could I do if I was a bad actor today?
In theory, by refining LLaMA with an array of harmful text and data, along with I/O from OpenAI's RLHF models, one could achieve performance comparable to GPT-4. Utilizing only OpenAI's models would likely not produce harmful text (given most of their outputs follow some safety standards).
I could then execute on many of the risks outlined by CIP here (e.g, poison the information sphere with easy-to create low-quality data and personalized disinformation).
2.2) The IP Paradox
LFM corporations stringently enforce prohibitions against replicating their models, yet they exploit public data for financial gain.
Many lawsuits continue to unfold, challenging the appropriation of public data by these LFM entities and the resulting profits. The resolution of these disputes remains unclear, raising open questions about the lack of compensation for individuals whose work has been appropriated by these LFM companies.
2.3) What does this mean?
On the positive side, this situation paves the way for decentralization in the AI domain, which is beneficial, as it prevents an exclusive reliance on say EA leaders for Sam Altman for AI development.
A negative perspective highlights the potential for empowering malicious actors to operate numerous detrimental LFMs, resulting in harmful consequences. LFM developers will have to strike a balance between maintaining accessibility and deterring rapid replication via datasets. Equally challenging is the task of democratizing access to these models.
Will there be prerequisites to obtain access to a model, and will these be equitable across various socio-economic backgrounds? What is the harm that is possible with open-source models combined with commercial I/O data, and what the optimal blend of open-source model and I/O data might be? What are the set of governance frameworks we can create to answer such questions for LFMs?
If you have work that addresses any of these questions, or are interested in working on answering them, email me shreyjaineth@gmail.com.
Readings for You
Ethics of Decentralized Social Technologies: Lessons from the Web3 Wave
GPTs are GPTs: An Early Look at the Labor Market Impact Potential of Large Language Models
Dissociating language and thought in large language models: a cognitive perspective
Large Language Models Fail on Trivial Alterations to Theory-of-Mind Tasks